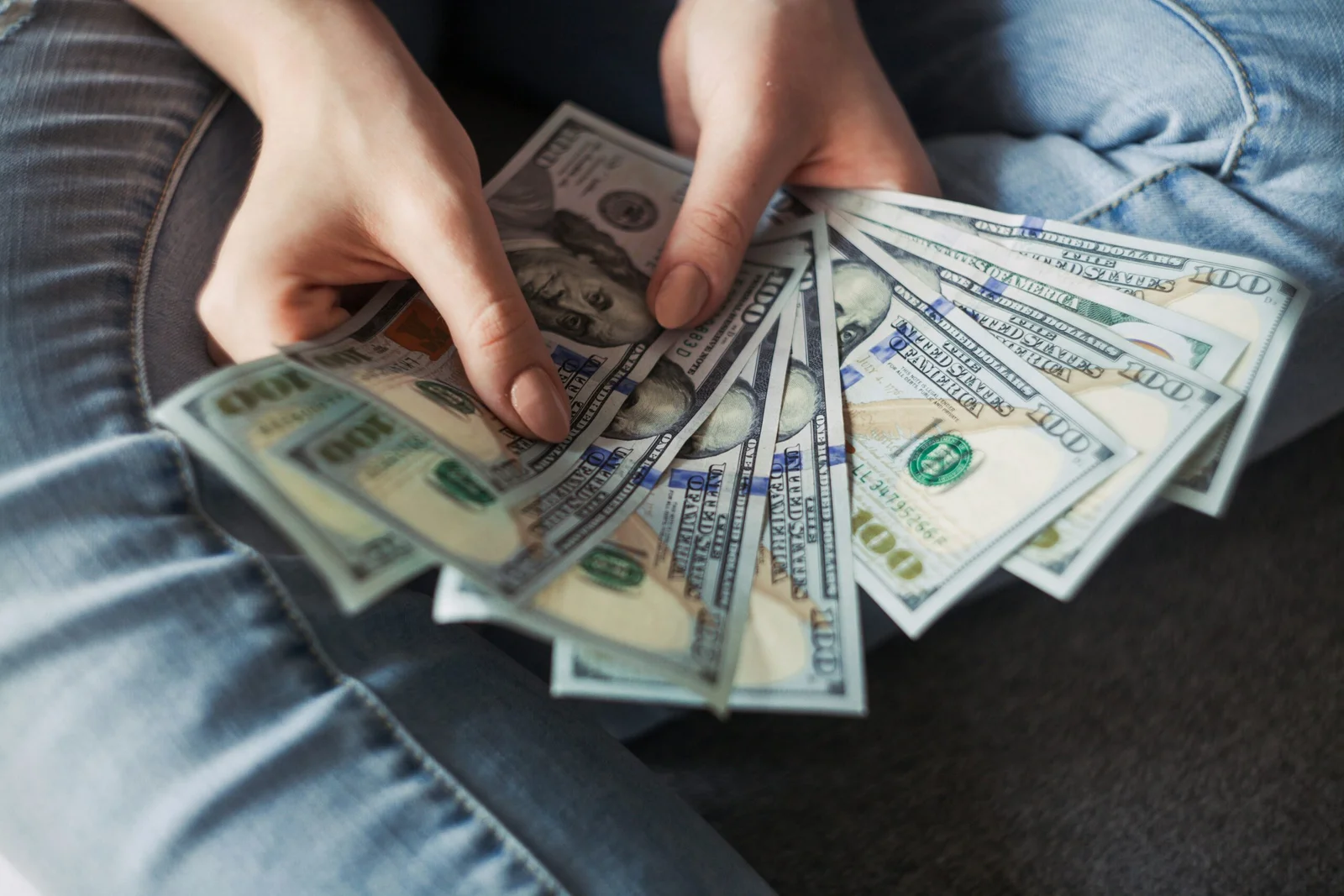
Data-Driven Decisions: Harnessing Technology for Better Banking Solutions
Introduction to Data-Driven Decision Making in Banking
Data-driven decision-making (DDDM) has emerged as a pivotal approach within the banking sector, enabling institutions to leverage information and analytics to guide their strategies and operational choices. By systematically analyzing large volumes of data, banks can enhance their decision-making processes, driving improvements in customer experiences, operational efficiency, and overall profitability. In an industry marked by rapid technological advancements and shifting consumer expectations, DDDM is no longer optional but essential for maintaining competitiveness.
The importance of data in banking cannot be overstated. With the rise of big data analytics, financial institutions can now gather and process vast amounts of information, ranging from transactional data to customer behaviors and preferences. This wealth of information allows banks to develop more personalized services, tailor marketing efforts, and create products that resonate with their clientele. For instance, by analyzing customer purchase patterns, a bank can offer targeted financial solutions that cater specifically to individual needs, thereby improving customer satisfaction and loyalty.
The banking landscape is undergoing a transformation driven by digital technologies and evolving consumer demands. Customers today expect seamless experiences, transparency, and instant access to information. In response to these trends, banks are compelled to innovate by incorporating DDDM into their operational frameworks. This commitment to data-centric practices not only supports enhanced decision-making but also fosters agility and responsiveness to market changes. As financial institutions strive to remain relevant, it is crucial to acknowledge that data-driven initiatives will be a cornerstone of their strategic approach.
Ultimately, the integration of technology and data analytics into the banking sector signifies a shift towards a more informed and proactive operational model. This paradigm not only enriches the customer experience but also positions banks to capitalize on emerging opportunities, ensuring long-term sustainability and growth in a rapidly evolving financial landscape.
The Role of Big Data in Banking
In the contemporary landscape of banking, the concept of big data has emerged as a critical element for driving efficiency and enhancing customer experience. Banks accumulate vast amounts of data from numerous sources, including transaction records, customer feedback, and market trends. This information can be categorized into two types: structured data, which consists of organized information such as transaction amounts and account numbers, and unstructured data, which includes insights from social media, customer interactions, and even machine-generated data. Harnessing both types of data enables financial institutions to develop a comprehensive understanding of their customers and the market.
The analytic capabilities afforded by big data empower banks to identify patterns within this wealth of information. For instance, by analyzing customers’ transaction histories, banks can discern spending habits and preferences. Such insights allow institutions to tailor their product offerings, ensuring that they align with the evolving needs of consumers. Furthermore, through predictive analytics, banks can anticipate shifts in consumer behavior, enabling proactive adjustments to their strategies. This capability is particularly valuable in an era where customer expectations are continually changing, demanding personalized and timely services.
Moreover, big data analytics enhances strategic decision-making within banks. By analyzing market trends and competitor performance, banks can identify emerging opportunities and risks, thereby making informed decisions that facilitate growth and mitigate potential threats. In this respect, big data acts as a powerful tool not only for understanding current market conditions but also for forecasting future developments. As the banking sector continues to evolve, the integration of big data into decision-making frameworks will become increasingly essential, paving the way for innovative banking solutions that are responsive to market demands.
Technological Tools for Data Analysis
The banking sector has witnessed a significant transformation due to the integration of advanced technological tools designed for data analysis. Prominent among these tools are artificial intelligence (AI) and machine learning (ML), which play a crucial role in automating data processing and enhancing predictive modeling capabilities. By employing AI algorithms, banks can analyze vast datasets more efficiently, allowing them to uncover trends and insights that would otherwise remain dormant.
Machine learning, a subset of AI, enables systems to learn from data patterns and improve over time without explicit programming. This capability is particularly beneficial for risk assessment, fraud detection, and customer relationship management within banks. For instance, machine learning models can predict customer behavior, helping banks tailor their services to meet client needs effectively. Furthermore, advanced analytics platforms provide robust frameworks for data visualization and interpretation, thus facilitating real-time decision-making.
The integration of these data analysis technologies into existing banking systems can streamline operations and foster a culture of informed decision-making. However, there are critical considerations concerning data security and privacy. As banks convert more data to digital formats and utilize cloud computing, they also become more susceptible to cyber threats. Therefore, implementing stringent data governance policies and established cybersecurity measures is paramount to protecting sensitive client information.
Moreover, compliance with regulations such as the General Data Protection Regulation (GDPR) is essential in ensuring that banks respect customer privacy while leveraging data for insights. Consequently, as banks continue to embrace advanced tools for data analysis, balancing innovation with security will be crucial for sustainable growth in the ever-evolving financial landscape.
Enhancing Customer Experience Through Data Insights
In the modern banking landscape, data-driven decision-making has become pivotal for enhancing customer experience. Banks utilize data insights to deliver personalized banking services, tailored to the specific requirements and preferences of their customers. By analyzing transaction data, customer demographics, and behavioral patterns, financial institutions can create targeted product offerings that resonate with individual clients, fostering a stronger connection between the bank and its customers.
One effective strategy employed by banks is the segmentation of their customer base. By categorizing customers according to their behaviors and preferences, banks can craft personalized marketing strategies that address the unique needs of each segment. For instance, a customer with frequent international transactions may receive tailored promotions on foreign exchange services, while a younger client may be targeted with mobile banking features and modern financial products. Such strategic use of data significantly enhances customer satisfaction and engagement levels.
Additionally, banks are increasingly utilizing customer feedback as a vital source of data for improving product offerings. By establishing feedback mechanisms—such as surveys, digital feedback forms, and social media engagement—banks can gather insights into customer sentiments and expectations. This data informs product development and service enhancements, allowing institutions to refine their offerings continually. A notable example can be observed with financial institutions that embraced user feedback to revamp their mobile banking apps, resulting in increased user adoption and satisfaction rates.
Furthermore, the implementation of advanced analytics tools enables banks to uncover patterns and predict future customer behavior, driving proactive customer service initiatives. By anticipating needs and addressing potential issues before they arise, banks can significantly enhance the overall customer experience, ultimately leading to increased loyalty and retention. In summary, leveraging data insights is essential for banks aiming to provide superior customer experiences, fostering lasting relationships built on trust and satisfaction.
Risk Management and Compliance Automation
The financial services sector, particularly banking, has experienced a paradigm shift towards data-driven decision-making, particularly in the realms of risk management and compliance automation. As banks are increasingly held to stringent regulatory standards, leveraging technology and data analytics becomes essential to meet these requirements effectively. By utilizing sophisticated data analysis tools, banks can identify potential risks, monitor transactions, and ensure compliance with regulatory frameworks that are often in flux.
One fundamental aspect of risk management in banking is detecting fraud. Traditional methods of fraud detection often fall short due to their reactive nature. However, with the integration of data analytics, banks can implement proactive risk management strategies. Advanced algorithms and machine learning techniques are now capable of analyzing vast amounts of transaction data in real time, enabling banks to identify unusual patterns that may indicate fraudulent activity. This proactive approach not only enhances security measures but also significantly reduces potential financial losses.
Transaction monitoring is another critical area where data analytics plays a crucial role. Banks employ automated systems that flag irregular transactions and automate the compliance reporting processes. These systems harness data from various sources, including transaction histories and customer profiles, to generate risk profiles. Consequently, by continually analyzing this data, banks can maintain vigilance over the activities that engage their systems, facilitating a quicker response to suspicious activities.
Moreover, understanding and analyzing risk factors is key to establishing a robust framework for risk management and compliance. By employing data-driven insights, banks can determine which areas of their operations present the greatest exposure to risk. This knowledge allows them to allocate resources more effectively and develop strategies that address specific vulnerabilities. Overall, the combination of data analytics with risk management provides banks with a clearer view of their operational landscape, ensuring they navigate the complexities of compliance more efficiently and effectively.
Operational Efficiency and Cost Reduction
The integration of data-driven decisions in banking has become increasingly vital for enhancing operational efficiency and facilitating cost reduction. By utilizing advanced data analytics techniques, banks can significantly optimize various processes, leading to improved service delivery while minimizing operational inefficiencies. One key aspect involves the careful examination of workflows to identify redundancies and bottlenecks that impede productivity. Through such analysis, banks can streamline their operations, ensuring that resources are allocated more effectively.
Resource allocation plays a pivotal role in achieving operational excellence. By leveraging data analytics, banks can analyze customer behavior, transaction patterns, and market trends to make informed decisions regarding the deployment of personnel and technological resources. This targeted approach can lead to significant cost savings, as banks are empowered to focus their investments on high-impact areas, ultimately enhancing service quality and customer satisfaction.
Several institutions have successfully adopted data-driven strategies, resulting in noticeable improvements in their operational efficiency. For instance, a notable case study involves a mid-sized bank that implemented predictive analytics to refine its customer service processes. By analyzing call volume and customer inquiries, the bank was able to optimize staffing schedules, which led to a 20% reduction in operational costs over a year. Another example can be seen in a large financial institution that harnessed data insights to automate routine processes, such as loan approvals and account management. This automation not only curtailed personnel-related expenses but also reduced transaction turnaround times significantly.
In summary, the strategic use of data-driven decisions is essential for banks seeking enhanced operational efficiency and reduced costs. By focusing on process optimization and resource allocation, financial institutions can eliminate redundancies and create a more streamline operation, positioning themselves for sustained success in a competitive marketplace.
The Future of Banking: Predictive Analytics and Beyond
The banking sector is on the cusp of a transformation fueled by advancements in data analytics and technology. As financial institutions increasingly rely on data-driven decisions, predictive analytics emerges as a pivotal tool. By processing vast amounts of data, banks can anticipate market shifts and customer behaviors more accurately than ever before. This capability allows institutions to tailor their services, improve customer experiences, and enhance risk management strategies based on empirical evidence.
Predictive analytics leverages historical data, statistical algorithms, and machine learning techniques to identify patterns and predict future outcomes. For instance, banks can forecast fluctuations in loan defaults or shifts in consumer spending habits. By analyzing customer transaction histories and demographic information, banks can segment their clientele and provide personalized product recommendations. Such capabilities not only improve customer satisfaction but also drive business growth through enhanced engagement and loyalty.
To stay competitive, banks must prioritize investments in technology that supports data analytics. This involves not only acquiring sophisticated tools but also fostering a culture of continuous improvement within their organizations. Training employees to interpret and utilize data effectively is crucial. Banks should encourage a mindset that values evidence-based decision-making, allowing teams to respond proactively to insights generated by predictive analytics.
Beyond predictive analytics, the future of banking will likely incorporate additional technologies such as artificial intelligence and artificial neural networks. These innovations can further enhance the capacity for data analysis, enabling banks to evaluate risks and opportunities in near real-time. As regulatory complexities and market dynamics evolve, embracing such technologies will be essential for financial institutions aiming to thrive in an increasingly data-centric environment.
Challenges in Implementing Data-Driven Solutions
The implementation of data-driven decision-making strategies within banking institutions often encounters several significant challenges. One of the primary issues is the existence of data silos, where information is stored in separate systems and is not easily accessible across departments. This fragmentation inhibits a bank’s ability to form a holistic view of its operations, customer behaviors, and market trends, ultimately limiting the effectiveness of data utilization. Breaking down these silos requires a cohesive data strategy that promotes interdepartmental collaboration and centralization of data resources.
Another considerable challenge is the skill gap present within many banking organizations. As the financial landscape continues to evolve with the integration of advanced technologies, existing staff might lack the necessary skills to effectively analyze and interpret data. This skill deficiency creates obstacles to the successful implementation of data-driven methodologies. To address this issue, banks must prioritize investment in employee training programs focused on data analytics and interpretation, equipping personnel with the needed competencies to leverage data effectively.
Additionally, resistance to change can be a considerable barrier when banks attempt to adopt data-driven solutions. Employees may be accustomed to traditional methods of operation and may view the shift toward data analytics with skepticism or fear. Managing this transformation requires robust change management strategies that highlight the benefits of data-centric workflows while providing ongoing support and encouragement throughout the transition period. By fostering a culture that values data-driven insights, banks can lessen resistance and cultivate an environment conducive to innovation.
In conclusion, the challenges associated with implementing data-driven solutions in banking are multifaceted, encompassing data silos, skill gaps, and resistance to change. By acknowledging these obstacles and developing targeted strategies, banks can pave the way for successful data-driven decision-making practices, ultimately leading to enhanced operations and improved customer service.
Conclusion: Embracing Data-Driven Decisions for a Competitive Edge
In the ever-evolving landscape of banking, the importance of data-driven decision-making cannot be overstated. As financial institutions face increasing competition and the challenges of a digital economy, leveraging technology and data analytics has emerged as a critical strategy for success. By integrating data into core operational processes, banks can enhance decision-making capabilities, offering personalized services that better meet customer needs.
Throughout this discussion, we have highlighted the myriad benefits that data-driven strategies provide for banks. From optimizing risk management to improving customer engagement, utilizing advanced analytics allows these organizations to derive insights that inform their operational and strategic decisions. Moreover, embracing technological innovations such as artificial intelligence and machine learning can lead to enhanced efficiency, reducing operational costs and reinforcing regulatory compliance.
Additionally, a data-centric approach fosters a culture of continuous improvement within banks. With access to real-time information, decision-makers can rapidly adapt to changing market conditions, ensuring that their institutions remain resilient and competitive. This adaptability is increasingly vital in a world where customer expectations are ever-shifting, placing pressure on banks to not only react but also proactively offer innovative solutions.
In light of these points, it is imperative for banks to prioritize and invest in data analytics as a foundational component of their operational strategy. This commitment to harnessing data analytics will not only improve the customer experience but will also result in better-informed business decisions overall. As the financial landscape continues to change, those banks that embrace data-driven decisions will undoubtedly secure a competitive edge. The call to action is clear: adopt innovative technology and strategic data management to thrive in today’s dynamic banking environment.