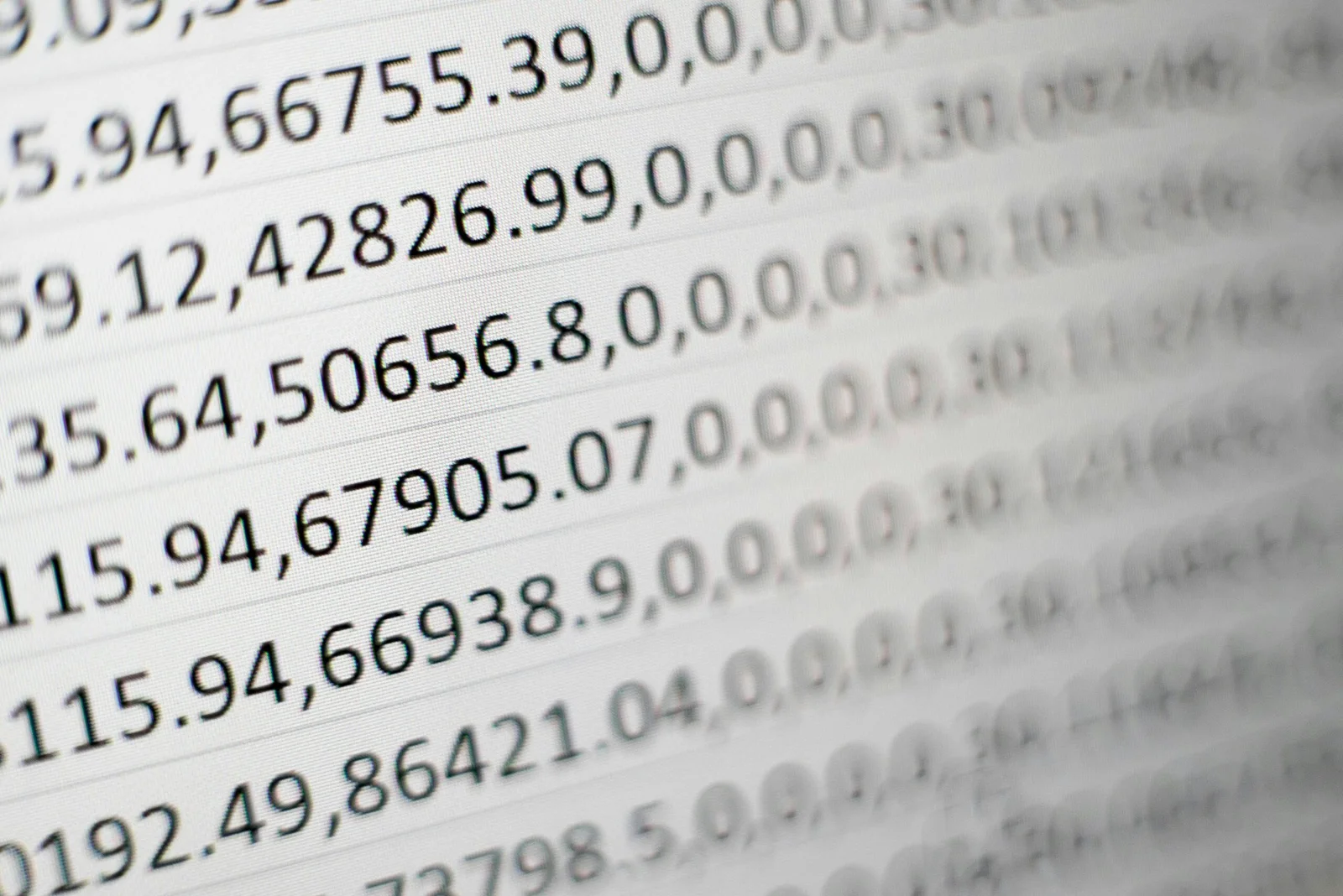
Harnessing Big Data for Better Farming Outcomes
Introduction to Big Data in Agriculture
Big data is increasingly becoming a pivotal force in various sectors, and agriculture is no exception. This term refers to the extensive volume of structured and unstructured data that can be analyzed for insights and informed decision-making. In the context of farming, big data encompasses a wide array of information, ranging from satellite imagery and sensor readings to historical crop yields and market trends.
The growth of technology plays a vital role in the agriculture sector, allowing farmers to collect and interpret vast datasets. The integration of Internet of Things (IoT) devices, such as soil moisture sensors and climate monitoring stations, enables the continuous gathering of real-time data. This information can be crucial for optimizing farming practices and ensuring sustainable agriculture. For instance, weather patterns can be tracked through historical data and current satellite imaging, which helps in making informed choices regarding planting and harvesting schedules.
Moreover, big data analytics provides farmers with valuable insights that drive efficiency and productivity. By analyzing patterns and trends, farmers can identify which crops are best suited for their land, what fertilizers yield the best results, and how to mitigate the risks associated with pests and diseases. Thus, big data serves as a powerful tool that empowers agricultural producers to make data-driven decisions, ultimately improving the overall yield and quality of the produce.
The increasing adoption of smart farming technologies illustrates the transformative nature of big data in agriculture. As farming practices evolve, the utilization of these vast amounts of data will likely become even more critical. This foundational understanding of big data’s role sets the stage for exploring its specific applications in enhancing agricultural outcomes.
The Role of Data Analytics in Farming
Data analytics has emerged as a transformative approach in modern farming, enabling agricultural stakeholders to leverage vast amounts of information for improved decision-making. By harnessing the power of data, farmers can optimize their operations, increase yields, and make informed choices regarding resource allocation. Various techniques within data analytics, including predictive modeling, machine learning, and data visualization, are at the forefront of this evolution.
Predictive modeling is a powerful tool that utilizes historical data to forecast future events. For instance, farmers can analyze past weather patterns, soil conditions, and crop performance to anticipate future yields. By understanding these trends, they can make strategic decisions about when to plant or harvest, thereby maximizing production. Similarly, machine learning algorithms can analyze complex datasets to identify patterns and correlations that may not be immediately apparent. This technology allows for real-time adjustments in farming practices, leading to higher efficiency and productivity levels.
Data visualization plays a crucial role in presenting complex datasets in an easily digestible format. By utilizing graphs, charts, and interactive maps, farmers can quickly interpret vast amounts of data, enhancing their ability to understand critical insights related to crop health, pest infestations, and irrigation needs. Clear visual representations empower decision-makers by facilitating the comprehension of data trends, enabling timely and accurate interventions.
Moreover, data analytics is instrumental in resource allocation, as farmers can assess the most efficient use of water, fertilizers, and pesticides based on analytical findings. This not only leads to cost savings but also promotes sustainable farming practices by minimizing waste and environmental impact. As the agriculture sector continues to evolve, embracing data analytics will be essential in achieving better farming outcomes and ensuring a food-secure future for communities worldwide.
Types of Data Utilized in Agriculture
The contemporary agricultural landscape is increasingly reliant on a diverse array of data types, which collectively inform farming strategies and decisions. Understanding these various categories of agricultural data is invaluable for optimizing farming outcomes. One of the most critical types is weather data, which includes temperature, precipitation, humidity, and wind speed. This data is typically gathered through meteorological stations, satellites, and weather apps. Farmers utilize weather forecasts to make informed decisions about planting, irrigation, and harvesting, thereby minimizing risks associated with adverse weather conditions.
Another essential category is soil health data, which encompasses various measurements, such as pH levels, nutrient composition, moisture content, and microbial activity. Technologies like soil sensors and lab analyses facilitate the collection of this data. By understanding soil health, farmers can implement tailored fertilizer applications and crop rotation strategies that enhance productivity while maintaining sustainability.
Crop health data is equally significant, as it provides insights into the condition of the crops throughout their growth cycle. This information is often gathered using remote sensing technologies, drones, and satellite imagery. Through the analysis of crop health data, farmers can detect issues such as disease, pest infestations, or nutrient deficiencies early, enabling timely and effective intervention strategies. Furthermore, monitoring crop health helps farmers increase yield quality and quantity.
Lastly, market trends data, which focuses on consumer preferences, pricing, and demand forecasts, plays a crucial role in determining which crops to plant. This information is often collected through market research, sales analysis, and customer feedback. By aligning farming decisions with market demands, farmers can mitigate the risk of surplus and ensure profitability. Each type of agricultural data contributes to a comprehensive decision-making framework, allowing farmers to adapt and thrive in a constantly evolving environment.
Technological Innovations Enabling Big Data in Farming
The integration of big data in agriculture is significantly driven by a range of technological advancements that facilitate the collection, analysis, and utilization of data. Key tools such as Internet of Things (IoT) devices have emerged as pivotal elements in modern farming. These connected devices allow for real-time monitoring of various environmental parameters including soil moisture levels, crop health, and atmospheric conditions. By harnessing IoT technology, farmers can make informed decisions based on accurate and timely data, leading to enhanced productivity and sustainability.
Another critical innovation augmenting big data capabilities in agriculture is drone technology. Unmanned aerial vehicles equipped with high-resolution cameras and sensors can cover large areas quickly, capturing detailed images and data that would be labor-intensive and time-consuming to collect on foot. This aerial imagery can be utilized for tasks such as crop health monitoring, pest detection, and precision application of fertilizers and pesticides. The data gathered through drones not only enhances the operational efficiency of farms but also contributes to informed decision-making processes.
Satellite imaging also plays a crucial role in the realm of big data for agriculture. With advancements in satellite technology, farmers can access detailed and accurate information about their fields over large geographical areas. This can include data on crop growth stages, soil erosion, and water stress. Such insights allow farmers to implement precise farming techniques, optimizing resource use while maximizing yield potential. Moreover, regular satellite assessments enable proactive management of potential issues before they escalate into significant problems.
Lastly, precision farming technologies are pivotal in the adoption of big data in agriculture. These solutions combine data analytics, machine learning, and geospatial technology to tailor farming practices to individual fields and even specific plants. By allowing farmers to apply inputs such as water, fertilizers, and pesticides in the exact amounts required, precision agriculture minimizes waste and enhances crop production efficiency. Together, these technological innovations form a robust framework that empowers farmers to harness big data effectively, ushering in a new era of agricultural productivity.
Case Studies of Successful Implementation of Big Data in Farming
In recent years, the agricultural sector has witnessed a transformative shift through the effective integration of big data technologies. Various farms and agricultural companies have embraced this trend, generating impressive results that underline the importance of data-driven strategies in improving farming outcomes. This section will explore several notable case studies that exemplify successful implementations of big data solutions in agriculture.
One prominent example is the case of a large corn and soybean farm in the Midwest United States. Faced with the challenge of inconsistent crop yields, the farm adopted a data analytics platform that collected and analyzed a variety of metrics, including soil quality, weather patterns, and crop health. By utilizing these insights, the farmers were able to optimize planting schedules and irrigation practices, leading to a remarkable 20% increase in yields over just one growing season. Additionally, the farm reported a significant reduction in input costs, thanks to more targeted resource allocation.
Another noteworthy case involves a vineyard in California, which faced challenges with pest management and disease control. By integrating big data solutions, the vineyard was able to employ precision agriculture techniques. Using drones equipped with advanced sensors, the vineyard monitored plant health and identified infestations with unprecedented accuracy. As a result, the vineyard saw a reduction in pesticide use by 30%, enhancing sustainability practices and improving the quality of the grapes harvested.
Similarly, a cooperative of dairy farms in Wisconsin implemented a big data system that tracked cow health and milk production metrics. By analyzing this data, the cooperative was able to identify early signs of illness in animals, allowing for timely intervention. This proactive approach resulted in a 15% increase in milk yield per cow and reduced veterinary costs by 25%, showcasing the powerful impact of big data analytics on livestock management.
These case studies illustrate the diverse applications and benefits of big data in agriculture. By tackling specific challenges through innovative data-driven strategies, farms and agricultural businesses have achieved remarkable outcomes, reinforcing the vital role of big data in modern farming practices.
Challenges in Leveraging Big Data for Agriculture
Farmers face numerous challenges when attempting to leverage big data for enhanced agricultural outcomes. One of the most prominent issues is data privacy. As farm operations become more digitized, the collection and sharing of sensitive information can raise concerns about unauthorized access and misuse of data. Farmers must navigate legal and ethical considerations while ensuring that their proprietary data is protected against potential breaches.
Another significant barrier to the effective use of big data in agriculture is the necessity for substantial infrastructure investment. Many farming operations, particularly smaller ones, may lack the necessary tools and technologies to collect, store, and analyze large datasets. Investments in reliable internet connectivity, advanced sensors, and robust data management systems are critical. However, the high initial costs associated with such upgrades can deter farmers from adopting big data techniques.
Furthermore, varied levels of technology adoption among farmers can create disparities in big data utilization. A segment of the agricultural community may be highly skilled at using technology, while others may have limited experience or knowledge. This variation can hinder collaborative efforts to analyze and leverage data effectively, thereby limiting the overall progress of data-driven practices in agriculture.
Data quality and integration represent another challenge in harnessing big data for agricultural applications. Inaccurate or inconsistent data can lead to erroneous conclusions, negatively impacting decision-making processes. Moreover, data from different sources often lacks standardization, making it difficult to integrate and interpret comprehensively. Ensuring the quality of data and finding ways to synchronize diverse datasets are essential steps for farmers aiming to benefit from big data analytics.
Future Trends in Big Data Agriculture
As we move further into the 21st century, the intersection of big data and agriculture is becoming increasingly prominent. The advancement of technologies such as artificial intelligence (AI), machine learning (ML), and automation is poised to fundamentally reshape farming practices. These technologies enable farmers to collect, analyze, and utilize vast amounts of data, improving decision-making and operational efficiency. For instance, AI-driven algorithms can process weather patterns, soil conditions, and crop health data to optimize planting schedules and resource allocation.
Machine learning algorithms are particularly noteworthy, as they can learn from historical data to predict future outcomes, thus allowing farmers to anticipate issues before they affect crop yields. This predictive analytics capability can help identify potential threats such as pest infestations or adverse weather conditions, ultimately contributing to enhanced crop resilience. Additionally, the integration of automation, including robotic systems and drones, empowers farmers to execute tasks such as planting, watering, and harvesting with minimal human intervention. This not only increases operational efficiency but also reduces labor costs.
Another critical aspect to consider is the evolving landscape of climate change and food security challenges. As global populations rise, the demand for food increases, necessitating innovative solutions to produce on a larger scale while preserving environmental sustainability. Big data applications play a vital role in addressing these issues by providing insights into resource management, precision agriculture, and sustainable practices. For example, data analytics can optimize irrigation practices to conserve water while maximizing crop output, thus responding to both productivity and environmental concerns.
In conclusion, the convergence of big data analytics with advanced technologies such as AI, machine learning, and automation heralds a new era for agriculture. By harnessing these innovations, farmers can navigate the complexities of climate change and food security, ensuring sustainable and efficient agricultural practices for the future.
Building a Data-Driven Culture in Agriculture
The agricultural sector is experiencing a transformative shift, particularly as advancements in technology continue to facilitate data utilization. To fully harness the potential of Big Data in farming, fostering a data-driven culture within agricultural organizations is of paramount importance. This cultural shift encourages farmers to integrate data analytics into their everyday operations, enabling them to make informed decisions that ultimately improve productivity and sustainability.
Education and training stand as essential components in promoting this data-driven mindset. By implementing comprehensive training programs focused on data literacy, farmers can become proficient in interpreting and leveraging data analytics. Such educational initiatives can involve workshops, online courses, and field-based training sessions that demonstrate the value of data in enhancing operational efficiency. Additionally, cultivating partnerships between academic institutions and agriculture-focused organizations can provide farmers with access to the latest research and methodologies in data analysis, further elevating their capabilities.
Collaboration plays a significant role in fostering a culture that prioritizes data utilization. When farmers collaborate with agricultural businesses and technology providers, they can share knowledge and best practices, facilitating mutual growth in data analysis competencies. Tech companies, in particular, have a critical role to play, offering tools and platforms that simplify data collection and interpretation for farmers. These collaborations can result in tailored solutions that meet the specific needs of farmers, ensuring that data-driven practices are accessible and applicable across various agricultural contexts.
As the agricultural landscape evolves, embracing a data-driven culture will be crucial for success. By investing in education, training, and collaboration, the farming community can significantly enhance its ability to harness Big Data, resulting in better farming outcomes and a more sustainable future for the industry. Encouragingly, as the awareness of the benefits of data analytics grows, it paves the way for wider acceptance and integration of these innovative practices in agriculture.
Conclusion: The Future of Farming with Big Data
As the agricultural landscape continues to evolve, the integration of big data into farming practices emerges as an undeniable catalyst for transformation. Throughout this blog post, we have explored the multitude of ways in which big data can enhance crop yields, streamline operations, and foster sustainability in agriculture. From predictive analytics that inform planting decisions to real-time monitoring systems that optimize resource usage, the applications of big data are extensive and varied.
Farmers and agricultural stakeholders are increasingly recognizing the importance of harnessing data analytics to make informed decisions. The use of precision farming techniques, supported by big data insights, can lead to more efficient use of resources such as water, fertilizers, and land. This approach not only helps to maximize productivity but also minimizes environmental impact, aligning with modern agricultural practices that prioritize sustainability.
The transformative potential of big data extends beyond individual farms; it has the capacity to reshape entire agricultural systems. By aggregating data from various sources, stakeholders within the agricultural sector can identify trends, forecast market demands, and improve supply chain efficiencies. This collaborative approach to data sharing can drive innovation and enable farmers to adapt to changing conditions while remaining competitive in the global marketplace.
It is essential for farmers and agricultural stakeholders to actively embrace the tools and technologies that big data offers. By investing in data-driven approaches, they can unlock new opportunities for efficiency and sustainability, enhancing the viability of the agricultural industry as a whole. The future of farming lies in the adroit use of data; thus, the call to action is clear: leverage the power of big data to forge a more prosperous and sustainable agricultural future.